How AI is disrupting our industry and what can we do about it? – this was the question Authority Magazin asked AI professionals and of course we were only too happy to talk about the changes, challenges and opportunities we see for AI in manufacturing.
The article is in form of an interview and you can find the complete version here.
I won’t lie, it’s a bit of a read and so here is a summary of the most important bits.
Can you explain how AI is disrupting your industry?
AI is the future of manufacturing. It is a powerful tool that is ideally suited to solve some of the more intractable issues that challenge manufacturers. The question is not whether manufacturers adopt AI, but when; will they do it sooner and get a competitive advantage, or will they wait to adopt until they are forced to in order to avoid their competitors leaving them in the dust?
Here are two examples from our own experience how AI is disrupting our industry:
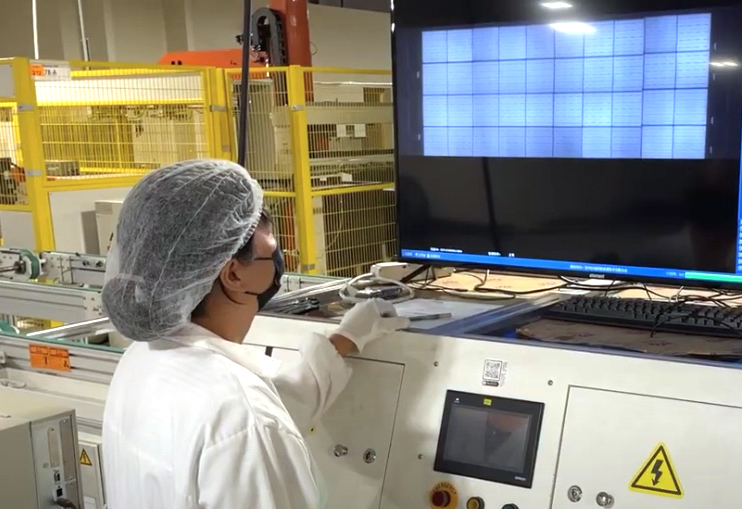
Quality control — the current methods of making sure products meet quality standards are often not up to the task: manual QC is slow, expensive, inaccurate and often completely infeasible. if you make 1,200 products a minute you can’t afford to hire people to check each one so you check samples at the risk of missing defective products. Even at lower volumes, people are generally not a good option: they are not good at focusing on a single task for 8 hours at a time, they get tired, make mistakes and often don’t enjoy their work. It is very hard to hire people for these repetitive jobs.
Traditional computer vision systems have other challenges: they are inflexible, every change in a product or a new product requires rewriting the code, the equipment is very expensive and still doesn’t meet high-speed requirements.
Enter AI; specifically, machine learning. These algorithms learn similarly to humans by seeing examples of good and defective products. Once trained properly they do their job 24/7/52 with consistent high accuracy. They learn to categorize defects, and even find whole new defect categories. They can adjust for environmental factors, such as different lighting conditions over the course of the day, and when you change product specs or add a new product all you need to do is retrain them with more images. In addition, they don’t require high end equipment, reasonably cheap cameras and sensors are good enough. This allows you to add quality stations at several points during production, not just at the end and remove defective products early before more time, material and energy are spent on them.
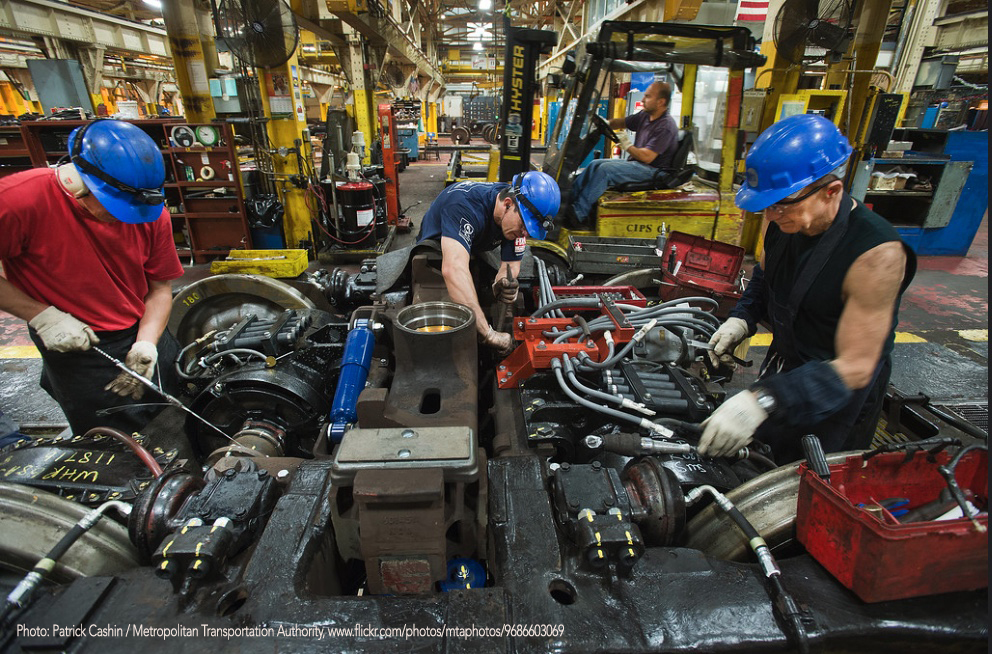
Predictive maintenance is another perfect use case for AI. If you feed the algorithms enough data about your critical equipment, such as pumps that are used to dispense a liquid and have a tendency to clog, add the information from existing maintenance records and let the AI do their pattern recognition magic, you will learn a lot about what causes unexpected failures. On a very practical note, the AI model can, based on its training, determine which pumps are most in need of maintenance and which ones don’t need attention. So now, instead of the maintenance crew routinely maintaining pumps 1 through 15 because it is the first Wednesday of the month, they can focus on those most likely to fail and not worry about the others.
This really helps streamlining maintenance and saves a lot of money because unplanned line downtimes caused by defective equipment can be very expensive.
We discuss two additional use cases in the Medium article.
Which specific AI technology has had the most significant impact on your industry?
If I had to single out AI technology with the biggest impact right now I would pick machine learning, especially deep learning algorithms. What makes these algorithms so powerful is the fact that they analyze data and learn to make predictions and decisions without being explicitly programmed. They can learn from past mistakes and are really good at processing images. The way they learn is similar to how a human learns: think of a child who learns to tell cats from dogs. You don’t provide them with lengthy definitions, you just tell them “Look, at the dog over there” and if they make a mistake, you tell them “This is a small dog, not a kitty”. Eventually the child will get it right every single time.
This is how AI learns to tell a good frozen pizza, tire rim, or concrete paver from one with too few slices of pepperoni, a scratch on the surface, or a missing corner.
What does it take to prepare the manufacturing workforce for the integration of AI, and what skills do you believe will be most valuable in an AI-enhanced future?
This is a hard nut to crack for manufacturers and at the same time it is an opportunity. Let’s face it, manufacturing is not particularly sexy, and as a manufacturer you simply can’t afford the salaries even fairly junior data scientists command and therefore buying AI expertise is not an option — you need to work with the talent you have, and build on their knowledge and expertise. Nobody knows products and processes better than employees, and therefore these employees need to be part of any AI implementation from day 1.
Building that expertise also provides exciting upskilling opportunities for existing employees and robust AI initiatives may help to make a company more desirable to job seekers.
Now, of course, you can’t just walk onto the shopfloor one day, round up five people and tell them that they now are in charge of AI. You have to make it feasible, even easy for people who are interested and ambitious to go up that learning curve. This is exactly where our company comes in, we have built the platform, wrote all the software needed to integrate AI with existing systems and have made it easy even for AI novices to learn how to train a model, deploy it to many lines and multiple plants and to coordinate what we believe eventually will be hundreds or even thousands of models.
What are your “Five Things You Need To Do, If AI Is Disrupting Your Industry”?
My top five things leaders in manufacturing, QC and operations need to do is:
1. Familiarize yourself with AI. There is a lot of hype out there and it is getting really difficult to follow everything that’s going on. The good thing is that you don’t have to, just focus on learning what AI can do for manufacturing, how it can help you solve concrete problems.
2. Give AI a chance to prove its value. Start slow, find an easy application — often quality control is a good starting point — and do a proof-of-concept study to demonstrate the value of AI to yourself and your colleagues. Nothing is as convincing as seeing AI in action.
3. Plan ahead to ensure success. You will need data, technology and people to make AI work. Most companies do not have the data they need so it needs to be collected. Technology is another area: you need the infrastructure to transmit images, enough disc space to store them, etc. People are possibly the hardest challenge; for example, you need IT/OT willing to work with you. I remember a project last year where IT dragged their feet on giving us remote access to their internal system for more than three months. When we finally got it, we had one week left to collect data before the seasonal project came to an end.
4. Don’t reinvent the wheel. While you want to develop AI skills in house and eventually be able to train and deploy your own models you don’t want to start at zero. Companies like ours have developed platforms that make integration with your internal systems, e.g. your PLCs and MES, very easy, and allow for quick deployment on many lines and across plants.
5. Don’t delay. I keep saying and I wholeheartedly believe that the question is not whether manufacturers will adopt AI but when they will do so. From where I stand it makes a lot of sense to get the process started now.
For more questions and answers and practical examples you really have to read the whole thing.
Did we pique your interest? Here is more for you to read: